By Zara Haider
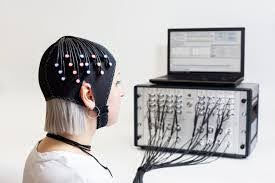
Cerebral blood flow is integral. Without it, serious medical consequences, such as strokes, can occur, which could lead to permanent damage or even death.
Strokes are a neurologic event where the brain is deprived of blood flow, which cuts off its oxygen and nutrient supplies. This can result in issues such as malfunction of speech, problem-solving, and even paralysis.
Conversely, enhanced blood flow supports brain function, allowing for greater physical and cognitive performance. A 1984 study conducted by researchers Fox and Raichle proved this with an observation of elevated levels of localized glucose and oxygen–delivered throughout the body via the vascular system– when subjects execute voluntary movements.
This dynamic fluctuation of cerebral blood flow and its polarizing effects can be monitored using Functional Near-Infrared Spectroscopy (fNIRS).
What’s fNIRS?
fNIRS is a neuroimaging technology that uses near-infrared light to indirectly monitor brain activity through hemodynamic flow. The technology measures levels of oxy and deoxyhemoglobin (oxygenated/deoxygenated blood) in the brain via light absorption.
Users put on a cross-hatched, flexible headset with near-infrared light emitters and sensors dotted all around. The headset is wired or wirelessly connected to computer software that records and compiles all the absorption rates at each sensor per unit of time, which doctors or researchers use to interpret a patient's cognition.
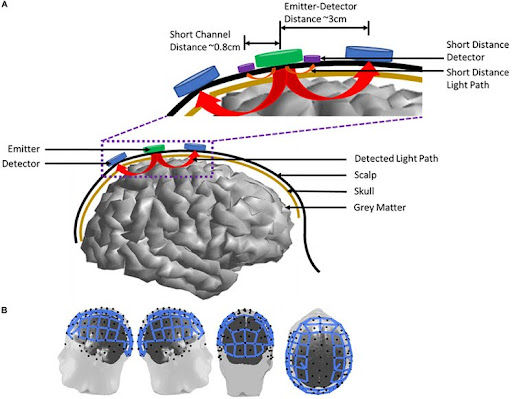
fNIRS provides the same measurement that its “slightly older cousin”, fMRI: blood oxygen level-dependent (BOLD) signals. BOLD detects changes in the ratio of deoxyhemoglobin (HHb) to oxyhemoglobin (O2Hb), which indicates the performance of a certain region of the brain. HHb absorbs near-infrared light at nanometers below 790, and O2Hb absorbs above that threshold. Changes in light absorption correlate to fluctuations in cerebral blood flow.
How fNIRS Surpasses fMRI
What’s fMRI?
Magnetic resonance imaging is a medical imaging technology that utilizes magnetic radio waves and 3D processing computer software to map out the human body.
Functional magnetic resonance imaging (fMRI) uses the same MRI machine but focuses on blood flow to the brain.
When a part of the brain switches from an inactive to an active state, the ratio of O2Hb to HHb becomes unbalanced, with an increase in HHb. This imbalance is corrected after the sphincter in the arterioles below the capillary bed releases O2Hb. The oxygen atoms break their bond with O2Hb to become HHb. fMRI detects this “recentering” as a change in the magnetic states of the blood.
HHb is paramagnetic, meaning that it produces a weakly attractive force towards the magnetic field created by the fMRI machine. The initial surge of HHb when a region of the brain becomes active causes the scan to show a brief dimming. Then, the diamagnetic O2Hb, which is the opposite of paramagnetic, rushes in and effectively brightens the area. This flash of light helps medical researchers easily monitor cognitive function.
Weaknesses of fMRI
Because MRI machines utilize magnetic fields to measure the magnetism of the hemoglobins, extra precautions must be taken to ensure that the subject and the equipment in the MRI machine room are magnet-safe. This can be an inconvenience if a patient has a magnetic implant or a metal hip replacement.
When a fMRI scan is taken, the ratio of O2Hb to HHb is recorded, but the individual quantities are not specified. Mathematically, this can give medical professionals an inaccurate picture of what’s going on. A normal BOLD ratio can mask the possibility of a patient having an abnormally low level of HHb, which could suggest a medical concern.
While a normal MRI scan takes 20-30 minutes, an fMRI scan takes 40-55 minutes and even longer if there are complications with the patient, equipment, software, or other external factors. This long duration can make fMRI scans of children and those with anxiety or ADHD especially difficult.
MRI machines have metal coils known as gradient coils which, after receiving an electric pulse, create a magnetic field for the computer software to detect the magnetism of O2Hb and HHb. That pulse vibrates the coil, causing a noise. These sounds can interrupt the near-infrared light from penetrating thoroughly, resulting in inaccurate results. Lastly, according to the University College of London, fMRIs cost ten times more than fNIRS scans, restricting accessibility to its services.
Strengths of fNIRS
fNIRS excels where fMRI falls short. Due to the use of near-infrared light, the absorption rate quantifies a patient’s O2Hb and HHb levels, allowing for a better understanding of the brain’s function.
The headset that patients wear allows movement, which can help scientists and medical professionals to have a better understanding of the relation between O2Hb and HHb under conventional activities.
The Setbacks of fNIRS
Despite fNIRS having certain benefits over fMRI, it does have its limitations.
fNIRS has a low spatial resolution, which makes it difficult for medical professionals to interpret a patient’s brain activity. This can hinder cognitive tests and research studies on seizures, as this fundamental feature of differentiating specific parts of the brain is crucial to determining which region is responsible for what. However, multichannel fNIRS has been made to mitigate this issue.
External noises and smaller sounds like heartbeat and head motions can distort or mask the near-infrared signal, thus hindering the technology’s ability to detect minute changes in the brain’s O2Hb/HHb levels.
Overcoming Noise Sensitivity
Scientists and medical professionals often use an fMRI scan in conjunction with a fNIRS to perform an enhanced evaluation.
To analyze fNIRS data the Fourier Transform, a mathematical tool, is applied to convert the time-domain data into the frequency domain.
Time domain data shows the changes in absorption of near-infrared light over time. It is also used to create a colorful graph for scientists and researchers to interpret and monitor fluctuations in brain activity.
The frequency domain breaks down the signal into frequency constituents, revealing how different frequencies contribute to the signal. From there, several methods, such as whitening and adaptive filtering, can be applied to amplify the signal made by the device and minimize interference. Focusing on the relevant frequency component produces a clear response from O2Hb and HHb.
Prewhitening is a technique used to remove rhythmic physiological noise, such as heart rate and breathing, from fNIRS data. This filter uses an autoregressive statistical model, which analyzes past data to predict future data, thereby removing any unexpected or irregular data points from the recorded feed. It is emphasized to minimize or eliminate any external disturbances made by the patient, such as head shaking or talking, as it can reduce this method’s effectiveness.
Adaptive filtering is a type of filter that adjusts itself to remove unwanted noise or errors in a signal. A 2018 study by Nguyen et al used this principle to improve the data collected for a finger-tapping task. They combined various data, including the expected brain responses, the signals emitted from nearby sensors, and other physiological noise (like heartbeat, and breathing) to create a filter that effectively reduced 77% of noise in O2Hb and 99% in HHb. However, this method demands careful handling. It needs well-defined parameters to understand what counts as noise, as it can produce biased results if it’s not managed correctly.
As with all technology, there is reliability and liability upon its use. As researchers and doctors collaborate and improve the deficiencies of fNIRS, it is likely to become a mainstream tool, like MRI and CT machines, for the general population.
Sources:
Chen, W.-L., Wagner, J., Heugel, N., Sugar, J., Lee, Y.-W., Conant, L., Malloy, M., Heffernan, J., Quirk, B., Zinos, A., Beardsley, S. A., Prost, R., & Whelan, H. T. (2020). Functional Near-Infrared Spectroscopy and Its Clinical Application in the Field of Neuroscience: Advances and Future Directions. Frontiers in Neuroscience, 14(724). https://doi.org/10.3389/fnins.2020.00724
Fox, P. T., & Raichle, M. E. (1984). Stimulus rate dependence of regional cerebral blood flow in human striate cortex, demonstrated by positron emission tomography. Journal of Neurophysiology, 51(5), 1109–1120. https://doi.org/10.1152/jn.1984.51.5.1109
Functional MRI of the Brain. (n.d.). Yale Medicine; Yale University. Retrieved September 14, 2024, from https://www.yalemedicine.org/conditions/functional-mri-imaging-the-brain#:~:text=A%20normal%20MRI%20of%20the
Haartsen, R., Jones, E. J., & Johnson, M. H. (2016). Human brain development over the early years. Current Opinion in Behavioral Sciences, 10, 149–154. https://doi.org/10.1016/j.cobeha.2016.05.015
Nguyen, H.-D., Yoo, S.-H., Bhutta, M. R., & Hong, K.-S. (2018). Adaptive filtering of physiological noises in fNIRS data. National Library of Medicine, 17(1). https://doi.org/10.1186/s12938-018-0613-2
Santosa, H. (2017, April). Characterization and correction of the false-discovery rates in resting state connectivity using functional near-infrared spectroscopy. ResearchGate. https://www.researchgate.net/publication/316860672_Characterization_and_correction_of_the_false-discovery_rates_in_resting_state_connectivity_using_functional_near-infrared_spectroscopy
UCL. (2018, January 29). Functional Near-Infrared Spectroscopy (fNIRS). UCL Psychology and Language Sciences. https://www.ucl.ac.uk/pals/research/speech-hearing-and-phonetic-sciences/shaps-research/functional-near-infrared-spectroscopy#:~:text=fMRI%20is%20very%20expensive%20compared
コメント